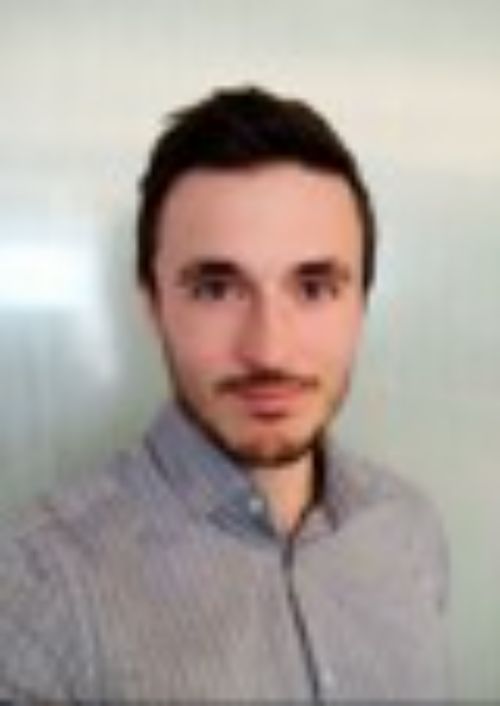
Ancestrality of genomic alterations in Multiple Myeloma: impact on the clonal evolution and on the Minimal Residual Disease dynamics
Funding Cycle:
2022-2023Name:
Andrea PolettiType of Award:
Career Development AwardHome Institution:
University of Bologna, ItalyHost Institution:
Dana-Farber Cancer InstituteDescription
Copy Number Alterations (CNAs) play a key role in the Multiple Myeloma (MM) pathogenesis and prognostic stratification of the disease. For this reason, it is of particular biological and clinical interest to study the temporal occurrence of early alterations over the developmental history of MM. This, in order to identify specific altered chromosomal regions or genes, which play a disease “”””driver”””” function by deregulating key tumor biological pathways. A correct identification of such alterations is especially important for the future development of a personalized medicine approach, which is aimed at identifying and targeting the specific biological “”””driver”””” alterations that characterize each individual tumor.
In this study an innovative suite of five bioinformatics tools (BOBaFit, RemasterCNA, RAPH, ComphyNumber and TestClonality) is developed. They were designed for the purpose of harmonizing Copy Number data and tracing the origin of CNAs throughout the evolutionary history of MM. To this aim, the largest available cohorts of newly diagnosed MM (NDMM) and Smoldering-MM (SMM) were aggregated, encompassing in total 1582 MMs and 282 SMMs collected from four different cohorts. This result was made possible by the collaboration of Prof. Irene Ghobrial’s laboratory from the Dana-Farber Cancer Institute of Boston.
The suite of tools enables the harmonization of CNA as obtained from multiple different genomic analysis platforms (e.g. WGS, WES, SNParray) in such a way that samples from the different cohorts can be merged and consequently a high statistical power of analysis can be obtained. By doing so, the high numerosity of those cohorts was harnessed for both 1) the identification (through the optimized use of the GISTIC tool) of novel of genes characterized as focal “”””driver”””” alterations in MM (including NFKB2, NOTCH2, MAX and EVI5 and MYC-ME2-enhancer genes), and 2) the generation of an innovative timing model based on the Bradley-Terry approach, implemented with the introduction of a statistical method to introduce statistical confidence intervals in the analysis of CNAs. This innovative model was developed after a careful review of the existing scientific literature in the field of temporal analysis of cancer, and it is capable of tracing quantitatively, in a confident and precise way, the events considered as “”””early”””” or primary in the evolutionary history of cancers.
By applying this model on both NDMM and SMM disease phases, it was possible to identify specific CNAs (amplification 1q(CKS1B), deletion 13q(RB1), amplification 11q(CCND1) and deletion 14q(MAX)) and categorize them as “”””early”””” and “”””driver”””” events with a high precision and confidence. This level of precision was guaranteed by the narrow confidence intervals in the timing estimates obtained. Thus, the identified CNAs were proposed as critical MM alterations, which play a foundational role in the evolutionary history of both SMM and NDMM. Importantly, among the identified events amp CKS1B and del RB1 were previously poorly characterized from an evolutionary point of view and uncertainly classified between primary and secondary events, while del MAX represent a completely new discovered MM driver alteration. Finally, a stepwise backward-forward Cox Regression survival model was able to optimally identify all the independent genomic alterations with the greatest effect on patients’ outcomes (Progression Free Survival and Overall Survival), including deletion of RB1, amplification of CKS1B, amplification of MYC, amplification NOTCH2 and deletion-mutation of TRAF3.
In conclusion, the alterations that were identified as both “”””early-drivers†and correlated with patients’ survival were proposed as new biomarker candidates that, if included in wider multivariate survival models, could provide a better disease stratification and an improved patient prognosis definition.